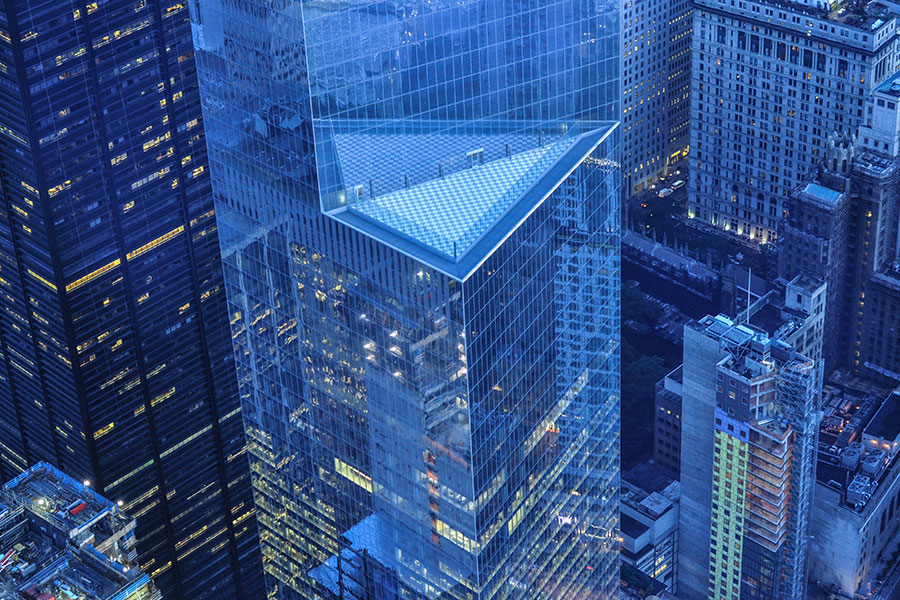
As Data is driving the next industrial revolution, businesses need to be increasingly seen as “maths” organisations such that those with the best algorithms that make the most accurate representations, inferences, analysis, predictions and recommendations are likely to dominate the market. But as market forces are changing at a rapid pace, hard coded algorithms may become outdated in a relatively short period of time. This opens up a space for the use of Machine Learning as it provides systems with “the ability to automatically learn and improve from experience without being implicitly programmed”
Several industries have great potential in leveraging the benefits of Machine Learning. Prominent among them is the Financial Services Industry which has been ranked at 4th place in McKinsey’s Digital Vortex. A research study by the Bank of England and the Financial Conduct Authority reveals that the Banking Industry remains by far the biggest consumer in the financial services industry for machine learning applications going forward. Typical use cases of Machine Learning in this sector include Compliance, Fraud Detection, Anti-Money Laundering, Loans and Credit Scoring, Customer Service, Stock Market as well as Risk Modelling in Investment Banking.
Success rates in transformation programmes involving the roll out of Machine Learning Applications however remain at best elusive. The Wall Street Journal Reports that 77% of executives interviewed claim that the business adoption of big data and artificial intelligence remains a major challenge. In fact, Gartner predicts that more than 85% of artificial intelligence projects will fail to deliver. So what could make these projects successful? Brock and Wangenheim (2019), sought to uncover the critical success factors for wider AI implementations through a research study involving more than 7,000 companies on a global scale. Their research uncovered seven common organisational characteristics amongst companies which had successfully implemented AI projects. These include integrated data management, CEO Priority, a mature security strategy, well defined digital processes, a clearly articulated digital strategy, strategic agility inbuilt in their operation and a culture of openness to innovation. Their findings resonate with Mckinsey’s conclusions that “successful transformation requires the development of a key capability we call digital business agility”.
But agility and the Banking Industry are seldomly used in the same phrase and for good reason. For starters, Banks are heavily regulated and any changes in their core processes may require some form of regulatory approval. Moreover, Brady (2018) suggests that digital transformation initiatives create “an explosion of cyber risk” as Banks are increasingly becoming prime targets for cybercrime. In Washington, the Federal Reserve has raised its eyebrows on the growing popularity of banks to implement machine learning solutions and has in fact called for more scrutiny by regulators. The Federal Reserve Governor has remarked that “the potential breadth and power of these new AI applications inevitably raise questions about potential risks to bank safety and soundness, consumer protection or the financial system.” (American Banker 2018). Amid safety concerns, Bank’s strong reliance on existing legacy systems are themselves creating barriers for the implementation of Machine Learning Solutions (Financial Conduct Authority).
In spite of “Agility” limitations posed by the Banking Sector, their highly data driven characteristics make them prime candidates to become “Cognitive Enterprises”. Indeed Solis (2020) posits that digital transformation has become “foundational” such that enterprises must “look beyond modernisation towards AI, machine learning and emerging technologies that are already starting to shape next-generation enterprises”. IBM defines Cognitive Enterprise as a company “that uses artificial intelligence and distributed technologies….to power and disrupt the markets of the future.” The foundations of cognitive enterprise requires an intersection of technology, culture and experience. Notwithstanding their high potential in leveraging return on investment from the implementation of Machine Learning Solutions as part of a process to reinvent themselves as Cognitive Enterprises, Banks are faced with a high risk of failure in these projects.
Whilst academic literature as well as practitioners have presented a plethora of generic frameworks for managing digital transformation programmes, it is believed that such frameworks do not address the specific requirements for implementing Machine Learning Systems. Failure rates remain high such that Organisations are left rudderless as to how to customise their management processes to minimise risk of failure. Indeed, such systems offer unique challenges which may be incompatible with other more traditional solutions that are available on the market. In the first instance, Machine Learning Systems are the lynchpin for the creation of the Cognitive Enterprise which requires a radical paradigm shift in an organisation’s strategy, structure, systems and processes. Moreover, it is further argued that in contrast to other digital transformation programmes which typically have a finite duration, the implementation of Machine Learning Systems require implementation support in perpetuity because “they never stop learning”. In fact it is further posited that whilst the value of traditional systems decrease over time due to technical obsolescence, the value of machine learning solutions appreciates exponentially as they accumulate experience and “learn” to perform their intended tasks in a better way. In this context, the true value that may be leveraged through machine learning solutions is not only dependent on the change management process put in place prior the “Go Live” date, but is highly contingent on the organisation’s capability to create a proper environment for these systems to “learn” to carry out their intended functions in a better way.
I am currently carrying out research with international banks in the UK and Europe with a view to design and develop a new and innovative transformation framework that can accommodate the specific requirements of applying Machine Learning Solutions in the Banking Industry. Please DM on [email protected] if you want to know more about our progress in this area.